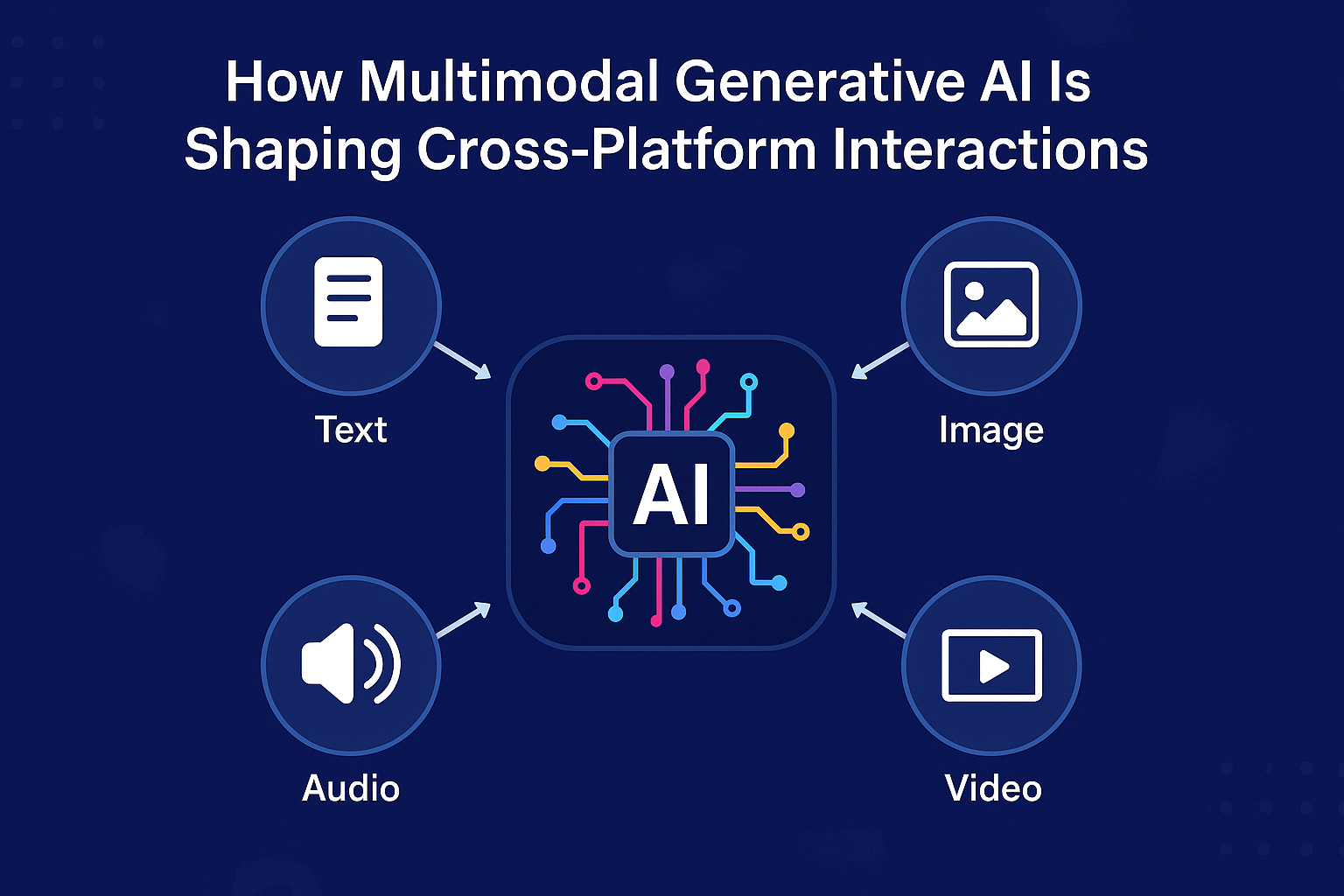
Artificial intelligence is advancing at breakneck speed, and one of its most transformative branches is multimodal generative AI. This technology has the unique ability to understand and process multiple data types—such as text, images, audio, and video—enabling it to generate contextually rich, highly relevant outputs. While its capabilities are game-changing, adopting and integrating multimodal AI across business platforms still poses practical challenges.
What Is Generative AI?
Generative AI is a type of artificial intelligence focused on creating new, original content. Unlike traditional AI models that simply analyze or react to inputs, generative AI can produce human-like outputs based on the patterns it has learned from extensive datasets.
-
It can generate diverse content types—text, images, audio, and video.
-
It is widely used in creative applications like design, writing, marketing, and art.
-
These models are trained on massive datasets to ensure realistic, high-quality outputs.
What Is Multimodal Generative AI?
Multimodal generative AI goes beyond traditional AI models by integrating various data types into one unified system. It processes and synthesizes inputs such as voice, visuals, and text to deliver more immersive and intelligent responses.
-
Multimodal AI model: Designed to handle multiple data formats simultaneously.
-
Multimodal AI systems: Leverage neural networks to draw meaningful correlations across modalities—like matching a spoken command to a visual output.
For example, a multimodal system can take a spoken query and an image and return a text-based solution that accurately responds to the entire context.
How Multimodal Generative AI Works
The inner workings of multimodal generative AI involve sophisticated algorithms capable of interpreting a variety of inputs and creating unified outputs. Here’s how it functions:
-
Input processing: The model can simultaneously interpret voice, text, images, or video.
-
Cross-modal training: Using large datasets that combine formats (e.g., pairing images with descriptive text), the AI learns how these modalities interact.
-
Context-aware outputs: Once trained, the model can generate responses that combine input sources. For instance, a user might send a photo and describe an issue verbally—the AI can then generate an accurate solution based on both.
Multimodal AI can radically improve customer interaction scenarios, especially where context is vital.
Real-World Use Cases of Multimodal Generative AI
From e-commerce to healthcare, multimodal generative AI is revolutionizing how companies operate and serve customers.
-
Retail and e-commerce: Virtual shopping assistants can understand voice queries and respond with visual product suggestions.
-
Customer service: AI systems can combine a customer’s spoken tone, textual message, and image uploads to provide nuanced support.
-
Contact centers: AI assistants can resolve issues faster by analyzing text inputs, voice tone, and product images simultaneously.
By processing a broader range of data, these systems make interactions feel more human, personalized, and responsive.
The Role of Multimodal Generative AI in Contact Centers
Contact centers are one of the biggest beneficiaries of this technology. Multimodal generative AI significantly boosts efficiency and customer satisfaction by enabling more intelligent, cross-format communication.
-
Integrated databases: AI systems now store and access text chats, voice logs, images, and even video interactions.
-
Smarter responses: These systems can learn from past interactions to adapt and improve future responses.
-
Holistic understanding: AI can handle queries across formats—for example, analyzing a customer’s voice while reviewing related chat logs and product photos.
This leads to more comprehensive, real-time solutions that reduce agent workloads while enhancing the overall customer experience.
The Future of Customer Support with Multimodal AI
As businesses seek to deliver exceptional customer service across more channels, multimodal AI will be central to their success. Its ability to provide responsive, intelligent, and context-aware interactions positions it as a foundational technology in the evolution of customer engagement.
-
Enables seamless communication across voice, text, image, and video.
-
Elevates support quality by interpreting customer sentiment and context holistically.
-
Offers personalized, scalable solutions for complex customer needs.
In the coming years, we can expect even more advanced systems that offer real-time transcription, emotional analysis, and multimodal personalization—all on a single platform.
Final Thoughts
Multimodal generative AI represents a significant leap in how businesses approach automation, personalization, and cross-channel communication. By merging multiple data types into a cohesive experience, it allows organizations to deliver superior customer service and build deeper engagement across every touchpoint.
Companies that adopt this technology early will be better equipped to meet evolving customer expectations, streamline operations, and differentiate themselves in a competitive landscape.
FAQs
1. What distinguishes Generative AI from traditional AI?
Generative AI specializes in creating new content—text, images, or music—based on learned data patterns. Traditional AI typically focuses on analyzing and responding to data rather than producing new outputs.
2. Is ChatGPT a multimodal model?
Currently, ChatGPT is primarily a text-based language model. While some newer versions have incorporated vision capabilities, traditional ChatGPT does not process audio or video.
3. Does ChatGPT qualify as adaptive AI?
To some degree, yes. ChatGPT adapts its responses based on contextual cues during a session. However, it does not learn continuously from user interactions in real-time.
4. Which models are more advanced than ChatGPT?
Models like GPT-4 with multimodal capabilities surpass ChatGPT in functionality. These can process and generate across formats, offering broader applications for AI-driven solutions.