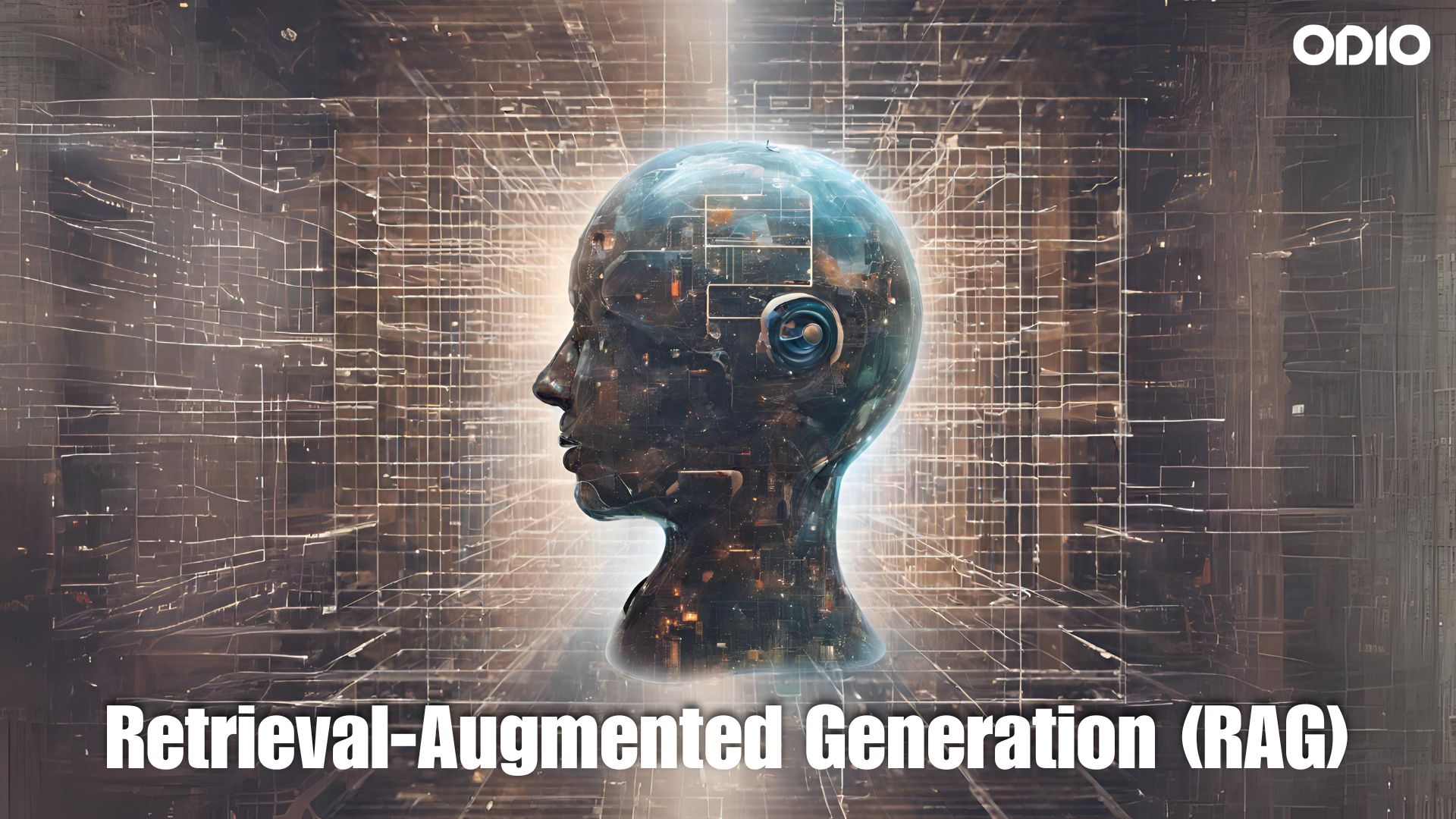
In the ever-evolving landscape of artificial intelligence, a new player has emerged to redefine the boundaries of information processing. Imagine a system that seamlessly combines the precision of retrieval with the creativity of generation, ushering in a new era of AI capabilities. This is the essence of RAG Retrieval-Augmented Generation, a cutting-edge technology poised to transform the way we interact with and generate content.
What is RAG Retrieval-Augmented Generation?
RAG, or Retrieval-Augmented Generation, is a powerful AI model that integrates the strengths of both retrieval and generation mechanisms. Unlike traditional language models that rely solely on generating content based on prompts, RAG incorporates a sophisticated retrieval system to pull in relevant information before generating responses. This dynamic synergy allows RAG to tap into a vast pool of knowledge and produce more contextually relevant and accurate content.
Why is RAG Retrieval-Augmented Generation Important?
The importance of RAG lies in its ability to bridge the gap between information retrieval and content generation, addressing key challenges in natural language understanding. In traditional models, generating content often lacks context, leading to inaccurate or incomplete responses. RAG, on the other hand, leverages its retrieval capabilities to access a diverse range of sources, ensuring that the generated content is not only contextually rich but also grounded in accurate information.
Consider a scenario where a professional is seeking in-depth information on a niche topic. Traditional models might struggle to generate content that goes beyond surface-level details. RAG, with its retrieval-augmented approach, excels in such situations by pulling in relevant data from a wide array of sources, enabling the generation of comprehensive and nuanced responses.
The importance of RAG becomes even more evident in domains where precision and context are paramount, such as legal, medical, and technical fields. Professionals in these areas can benefit significantly from the enhanced capabilities of RAG, streamlining their workflow and ensuring the accuracy of generated content.
As we delve deeper into the mechanics of RAG, its role in reshaping the landscape of information processing becomes increasingly apparent. The potential applications are vast, ranging from content creation and knowledge synthesis to real-time decision support systems.
How does Retrieval-Augmented Generation Work?
The magic behind RAG lies in its innovative approach to combining retrieval and generation. When presented with a prompt, RAG first employs a retrieval mechanism to identify and extract relevant information from a vast pool of pre-existing knowledge. This retrieval step ensures that the generated content is not isolated but grounded in a contextual understanding of the subject matter. The retrieved information serves as the foundation upon which the generation module builds, allowing RAG to produce more nuanced, accurate, and contextually rich responses.
The retrieval process itself relies on advanced techniques, often utilizing pre-trained language models and semantic search algorithms. This ensures that RAG can efficiently navigate through a multitude of sources, ranging from scientific literature and databases to news articles and online forums. The result is a holistic understanding of the topic at hand, empowering RAG to deliver content that goes beyond the limitations of traditional language models.
Benefits of Retrieval-Augmented Generation
Enhanced Context and Precision:
RAG’s ability to retrieve relevant information enables it to generate content with a level of context and precision that surpasses traditional models. This is particularly valuable in fields where accuracy is paramount, such as legal documentation, medical diagnoses, and technical documentation.
Efficient Knowledge Synthesis:
Professionals across diverse domains can leverage RAG to synthesize knowledge from various sources, streamlining research processes and decision-making. The technology acts as a knowledge assistant, providing comprehensive and well-informed responses in real-time.
Improved Content Generation:
Content creators benefit from RAG’s capabilities to produce high-quality, contextually relevant content. Whether drafting articles, reports, or marketing materials, RAG enhances the creative process by incorporating accurate and diverse information.
Real-time Decision Support:
In dynamic environments where decisions must be made rapidly, RAG’s retrieval-augmented approach ensures that generated responses are not only quick but also well-informed. This makes it a valuable tool in scenarios such as customer support, legal analysis, and financial forecasting.
The amalgamation of retrieval and generation in RAG opens new possibilities across industries, transforming the way professionals access information and create content. As organizations embrace this technology, the impact on productivity, accuracy, and innovation is expected to be profound.
Conclusion: The Future of Retrieval-Augmented Generation
In conclusion, the advent of RAG Retrieval-Augmented Generation marks a paradigm shift in information processing. The seamless integration of retrieval and generation not only enhances the accuracy and context of content but also opens avenues for unprecedented applications across diverse fields. As we stand on the cusp of a new era in AI-driven technologies, the future of Retrieval-Augmented Generation holds promises of more efficient workflows, enhanced decision-making, and a deeper synthesis of knowledge.
As we witness the transformative power of RAG, one can’t help but wonder: What possibilities will arise when retrieval and generation converge even further? How will RAG evolve to meet the evolving needs of professionals in various domains? The journey into the future of information processing continues, and RAG is leading the way.
Thank you for reading. We hope this article has been helpful in providing you with valuable information. For more insightful blogs like this, please follow our blogs at Odio.